AI-Generated "Poop" Podcast: Extracting Meaning From Repetitive Documents
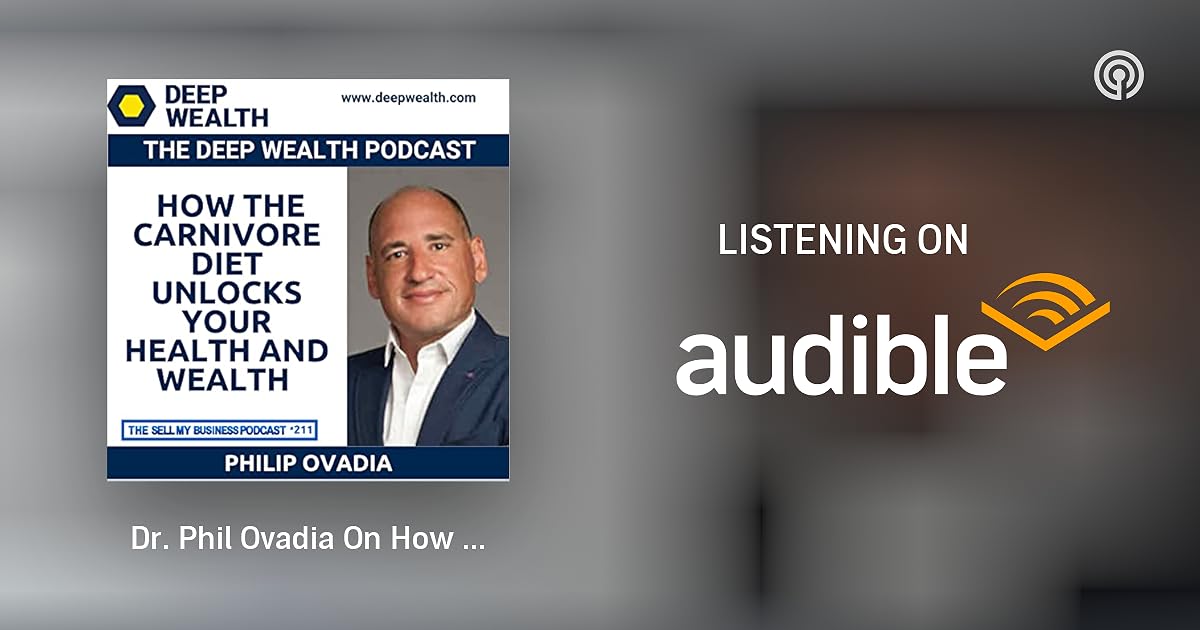
Table of Contents
Identifying the "Poop": Recognizing Repetitive Data Patterns
What exactly constitutes repetitive data? It's the kind of data that seems redundant at first glance, but holds hidden patterns and connections. Think:
- Endless Meeting Minutes: Similar discussions, recurring action items, and predictable outcomes across numerous meetings.
- Financial Reports: Consistent data structures, recurring key performance indicators (KPIs), and predictable trends.
- Legal Documents: Repetitive clauses, standard legal language, and similar case details.
- Customer Service Transcripts: Recurring customer complaints, frequent questions, and common issues.
Manually analyzing this type of repetitive data is a nightmare. It's incredibly time-consuming, prone to human error, and often misses crucial patterns buried within the mass of information. However, by employing specific techniques, we can effectively identify these repetitive patterns:
- Keyword Frequency Analysis: Identifying words and phrases that appear most often to uncover dominant themes.
- N-gram Analysis: Examining sequences of words to identify frequently occurring phrases and collocations.
- Topic Modeling: Uncovering latent semantic structures and themes within large volumes of text.
- Duplicate Detection: Identifying and removing exact or near-duplicate documents to streamline analysis.
Tools like Python libraries such as NLTK and spaCy can significantly aid in this process, offering powerful functionalities for text processing and pattern identification.
AI-Powered Techniques for Meaningful Extraction
The magic happens when we leverage the power of Artificial Intelligence, specifically Natural Language Processing (NLP). NLP allows computers to understand, interpret, and process human language. This opens up a world of possibilities for extracting meaningful insights from repetitive data. Key NLP techniques include:
- Sentiment Analysis: Gauging the overall sentiment (positive, negative, or neutral) expressed within documents, invaluable for understanding customer feedback or market sentiment.
- Named Entity Recognition (NER): Identifying and classifying named entities like people, organizations, locations, and dates, vital for extracting key players and contextual information.
- Relationship Extraction: Uncovering relationships between entities, providing insights into connections and dependencies within the data.
- Summarization: Generating concise summaries of large volumes of text, saving valuable time and providing high-level overviews.
Furthermore, machine learning algorithms play a critical role. Clustering algorithms can group similar documents together, while classification models can categorize documents based on pre-defined criteria.
Transforming "Poop" into Actionable Insights: Real-World Applications
The practical applications of AI-powered text analysis on repetitive data are vast. Let's look at some examples:
- Case Study 1: Customer Service Optimization: Analyzing customer service transcripts reveals recurring issues, allowing companies to proactively address customer concerns and improve satisfaction.
- Case Study 2: Research Synthesis: Extracting key findings from numerous research papers streamlines literature reviews, enabling researchers to create comprehensive reports efficiently.
- Case Study 3: Financial Forecasting: Identifying trends and patterns in financial reports aids in better investment decisions, risk management, and financial planning.
The benefits are undeniable: significant cost savings, increased efficiency, improved accuracy, and the discovery of previously hidden insights – all achieved by turning "data poop" into actionable intelligence.
Choosing the Right Tools and Technologies
Numerous AI-powered text analysis tools and platforms are available, each with its own strengths and weaknesses. Consider factors like:
- Budget: Free and open-source tools exist alongside enterprise-level solutions.
- Data Volume: The scale of your data will influence the processing power required.
- Technical Expertise: Some tools require significant programming skills, while others offer user-friendly interfaces.
- Specific Needs: Different tools excel at specific NLP tasks (e.g., sentiment analysis, summarization).
Remember, data preprocessing and cleaning are crucial steps before applying any AI techniques. Garbage in, garbage out, as they say. Furthermore, ethical considerations must be addressed, ensuring data privacy and responsible AI usage.
Conclusion: Turning Data "Poop" into Gold with AI
AI-powered text analysis offers a revolutionary approach to managing and interpreting repetitive data. By transforming seemingly useless "data poop" into actionable insights, organizations can gain a competitive edge, improve efficiency, and make better decisions. Don't let your data poop go to waste – unlock valuable insights with AI-powered text analysis today! Start exploring available tools and technologies, and begin turning your repetitive documents into valuable assets.
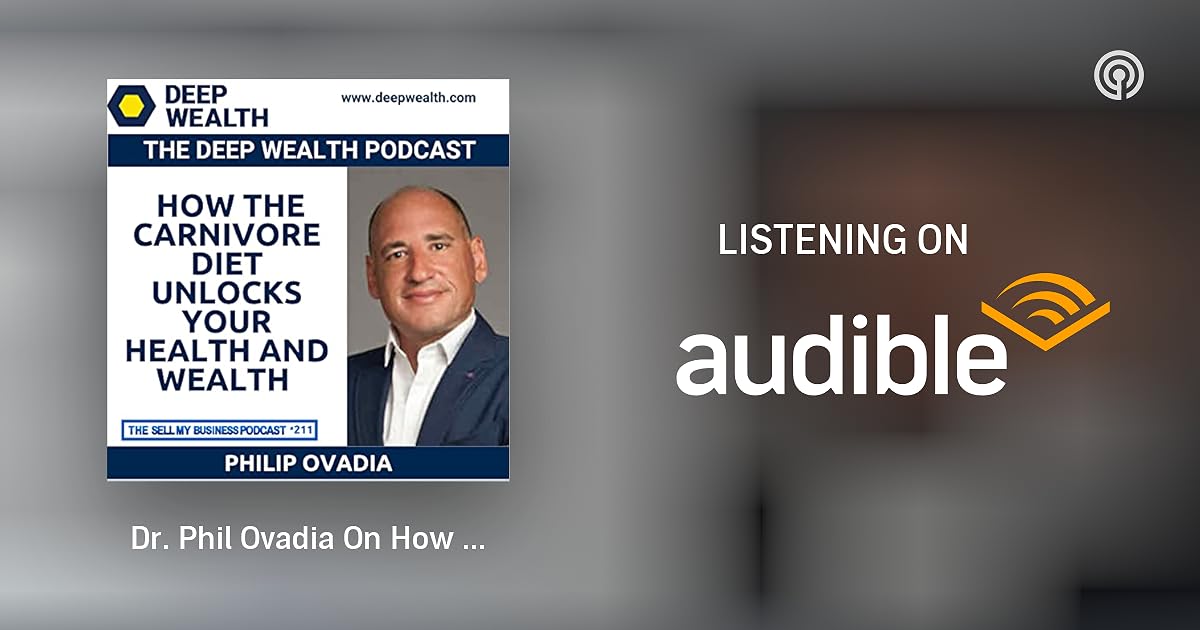
Featured Posts
-
Bekam Zarem Ima Konkurentsi A
May 09, 2025 -
9 Maya Zelenskiy I Ego Mezhdunarodnaya Izolyatsiya
May 09, 2025 -
Suspected Madeleine Mc Cann Impersonator Apprehended In Uk
May 09, 2025 -
The Best Victim In High Potential Season 2 A Case For Character Name
May 09, 2025 -
Stock Market Update Sensex And Nifty 50 End Choppy Trading Session Flat
May 09, 2025