Exploring The Surveillance Capabilities Of AI In Mental Health Treatment
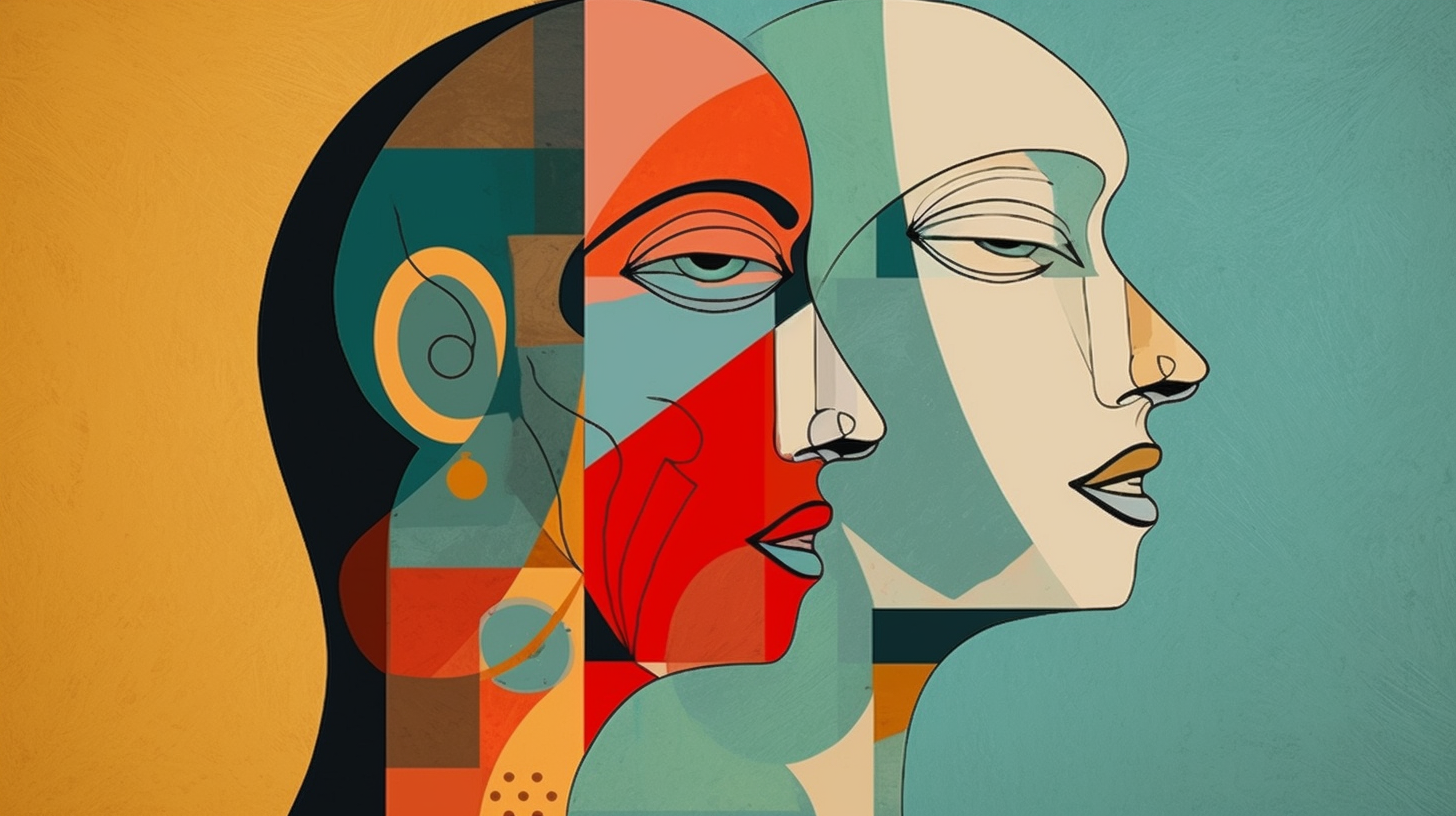
Table of Contents
AI Tools and Their Surveillance Potential in Mental Healthcare
The integration of AI into mental health is rapidly expanding, utilizing various tools with inherent surveillance capabilities. Understanding these capabilities is crucial for navigating the ethical landscape.
Wearable Sensors and Continuous Monitoring
Wearable devices, such as smartwatches and fitness trackers, collect physiological data like heart rate variability, sleep patterns, and activity levels. This data can be analyzed by AI algorithms to detect subtle shifts indicative of worsening mental health conditions, potentially enabling early intervention and relapse prevention. For example, increased heart rate variability at night might suggest rising anxiety, prompting proactive support. However, the continuous monitoring raises serious privacy concerns.
- Examples of specific wearable technologies: Fitbit, Apple Watch, Oura Ring, specialized biosensors.
- Data storage and security protocols: Secure cloud storage, encryption, anonymization techniques are crucial but not always foolproof.
- Potential for misuse of data: Unauthorized access, data breaches, and potential for insurance discrimination are significant risks.
Smartphone Apps and Data Collection
Numerous mental health apps collect vast amounts of user data, including mood entries, therapy session notes, location data, and social media interactions. AI algorithms analyze this data to personalize treatment plans, identify triggers, and monitor progress. While this personalized approach offers significant benefits, the continuous collection of such intimate data raises ethical questions.
- Examples of popular mental health apps: Woebot, Calm, Headspace, BetterHelp.
- Types of data collected: Mood ratings, symptom tracking, sleep patterns, medication adherence, GPS location (often optional).
- Potential for algorithm bias and discrimination: Algorithms trained on biased datasets may perpetuate existing health disparities.
AI-Powered Chatbots and Conversational Monitoring
AI-powered chatbots provide readily accessible mental health support, offering 24/7 availability and anonymity. These chatbots analyze user conversations to detect patterns, keywords, and emotional cues indicating potential mental health crises. While this facilitates early intervention, the storage and analysis of sensitive conversational data pose significant privacy risks.
- Examples of AI chatbots used in mental health: Woebot, Youper, Koko.
- Data encryption and security measures: End-to-end encryption and robust security protocols are essential to protect user privacy.
- Potential for misinterpretation of user input: AI algorithms may misinterpret nuances in language, leading to inaccurate assessments or inappropriate responses.
Ethical Considerations and Data Privacy in AI-Driven Mental Health
The application of AI in mental health necessitates a robust ethical framework to protect patient rights and ensure responsible innovation. Key considerations include:
Informed Consent and Patient Autonomy
Obtaining truly informed consent for data collection and use is paramount. Patients must fully understand the implications of AI surveillance, including data sharing practices and potential risks. However, ensuring comprehension of complex AI systems can be challenging, particularly for individuals experiencing mental health distress.
- Best practices for obtaining informed consent: Clear, concise language, accessible formats, opportunities for questions and clarification.
- Legal frameworks governing data privacy in healthcare: HIPAA (US), GDPR (EU), and other regional regulations.
- Challenges in ensuring patient understanding of complex AI systems: The need for simplified explanations and patient-friendly materials.
Data Security and Protection against Breaches
Mental health data is extremely sensitive. Robust security measures are essential to protect against breaches and unauthorized access. Data encryption, secure storage, and regular security audits are crucial. The consequences of a data breach can be devastating, potentially leading to identity theft, reputational damage, and discrimination.
- Data encryption techniques: AES-256, RSA, and other strong encryption algorithms.
- Security protocols for data storage and transmission: Multi-factor authentication, intrusion detection systems, regular security audits.
- Regulatory compliance standards: HIPAA, GDPR, and other relevant data privacy regulations.
Bias in AI Algorithms and Algorithmic Fairness
AI algorithms trained on biased datasets can perpetuate existing inequalities in mental healthcare. For example, algorithms trained primarily on data from one demographic group may fail to accurately diagnose or treat individuals from other groups. Addressing algorithmic bias requires careful data collection, diverse development teams, and rigorous testing.
- Examples of potential biases in AI algorithms: Racial, gender, socioeconomic biases.
- Strategies for mitigating bias: Careful data curation, algorithmic auditing, fairness-aware machine learning techniques.
- The role of diversity and inclusion in AI development: Diverse teams are crucial for creating fairer and more equitable AI systems.
Balancing Innovation with Patient Rights: A Path Forward
Navigating the ethical complexities of AI in mental healthcare requires a multi-faceted approach:
Regulatory Frameworks and Policy Recommendations
Clear regulatory frameworks are essential to govern the use of AI in mental health, balancing innovation with patient protection. These frameworks should address data privacy, algorithmic fairness, informed consent, and accountability.
- Examples of existing regulations related to AI and healthcare: HIPAA, GDPR, and emerging national and international regulations.
- Proposed changes to existing regulations: Strengthening data privacy protections, establishing clear guidelines for algorithmic transparency and accountability.
- The need for international cooperation on AI ethics: Global collaboration is essential to develop consistent standards for ethical AI development and deployment.
Transparency and Explainability in AI Systems
Transparency in AI algorithms is crucial for building trust and accountability. Explainable AI (XAI) techniques should be employed to allow patients and clinicians to understand how AI systems make decisions. This understanding empowers patients and promotes shared decision-making in their care.
- Techniques for enhancing transparency in AI systems: Model interpretability techniques, clear documentation of algorithms and data sources.
- The development of XAI tools: Tools that provide clear and understandable explanations of AI decisions.
- The benefits of patient education on AI in mental health: Empowering patients to understand and participate in their care.
Conclusion
The surveillance capabilities of AI in mental health treatment offer significant potential benefits, but these must be carefully weighed against the considerable ethical challenges. The responsible development and deployment of AI in this sensitive field requires a commitment to robust data security, transparent algorithms, informed consent, and algorithmic fairness. The future of AI in mental healthcare hinges on our commitment to ethical development and responsible implementation. Let's continue the conversation on how we can harness the power of AI while upholding patient privacy and ensuring equitable access to care. Further research and discussion on the surveillance capabilities of AI in mental health treatment are crucial for shaping a future where technology empowers, rather than endangers, vulnerable individuals.
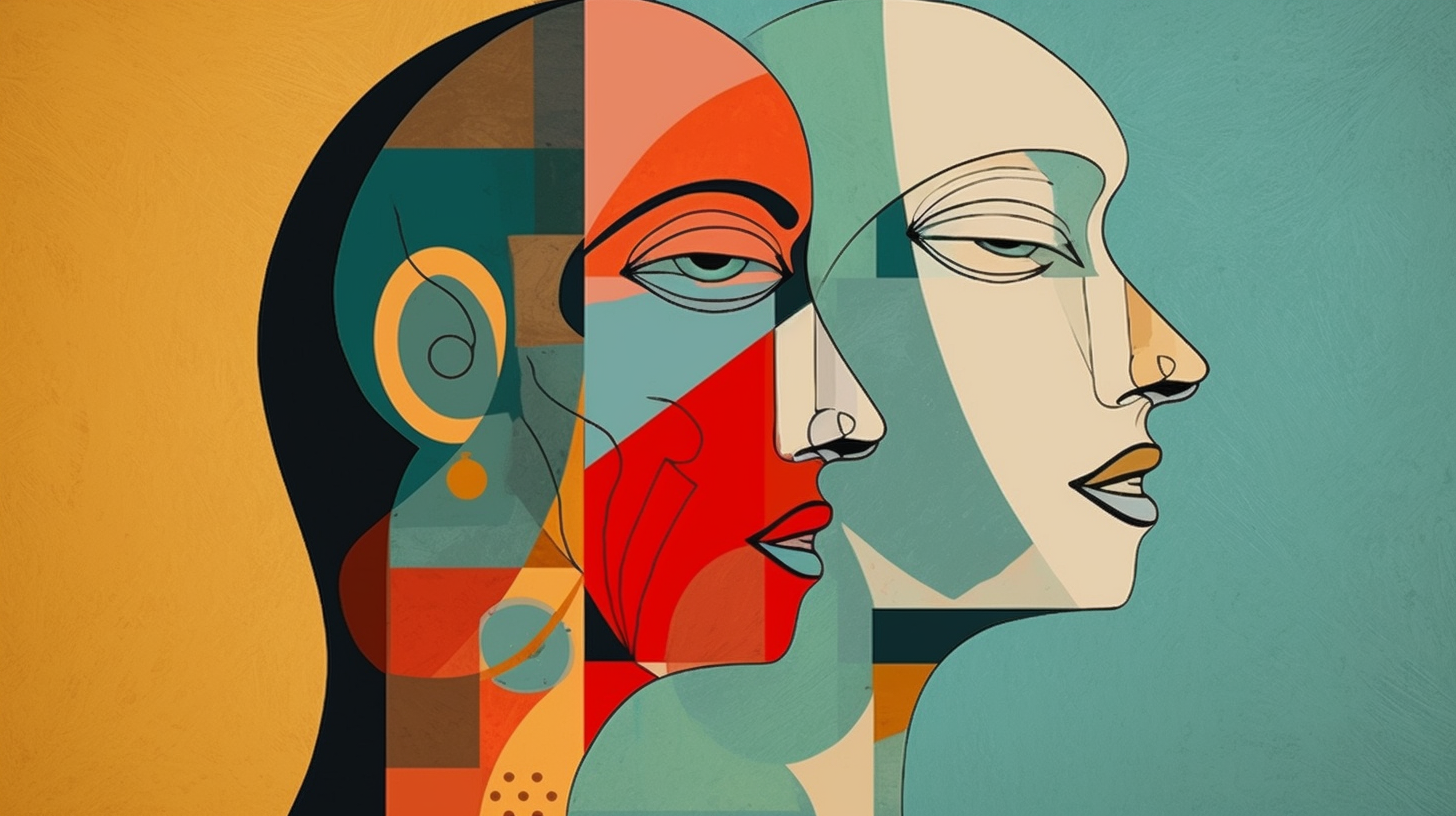
Featured Posts
-
La Liga Hyper Motion Almeria Eldense En Directo Online
May 16, 2025 -
Heat Butler Rift Jersey Numbers Highlight Deep Seated Tension Hall Of Famer Involved
May 16, 2025 -
Ecuador To Charge Correas Former Vp In Candidates Murder
May 16, 2025 -
Obstrel Ukrainy Analiz Masshtabnoy Ataki Rf 200 Raket I Dronov
May 16, 2025 -
Almeria Eldense Ver El Partido En Directo Por La Liga Hyper Motion
May 16, 2025
Latest Posts
-
Vont Weekend 2025 A Photo Journal April 4 6 107 1 Kiss Fm
May 16, 2025 -
1 Kissfms Vont Weekend A Photo Journal April 4 6 2025
May 16, 2025 -
Smart Mlb Dfs Plays For May 8th Sleeper Picks And Hitter To Bench
May 16, 2025 -
1 Kiss Fms Vont Weekend Photo Highlights April 4 6 2025
May 16, 2025 -
Mlb Dfs May 8th Proven Sleeper Picks And Player To Exclude
May 16, 2025