I/O Vs. Io: The Future Of AI Development And The Google-OpenAI Rivalry
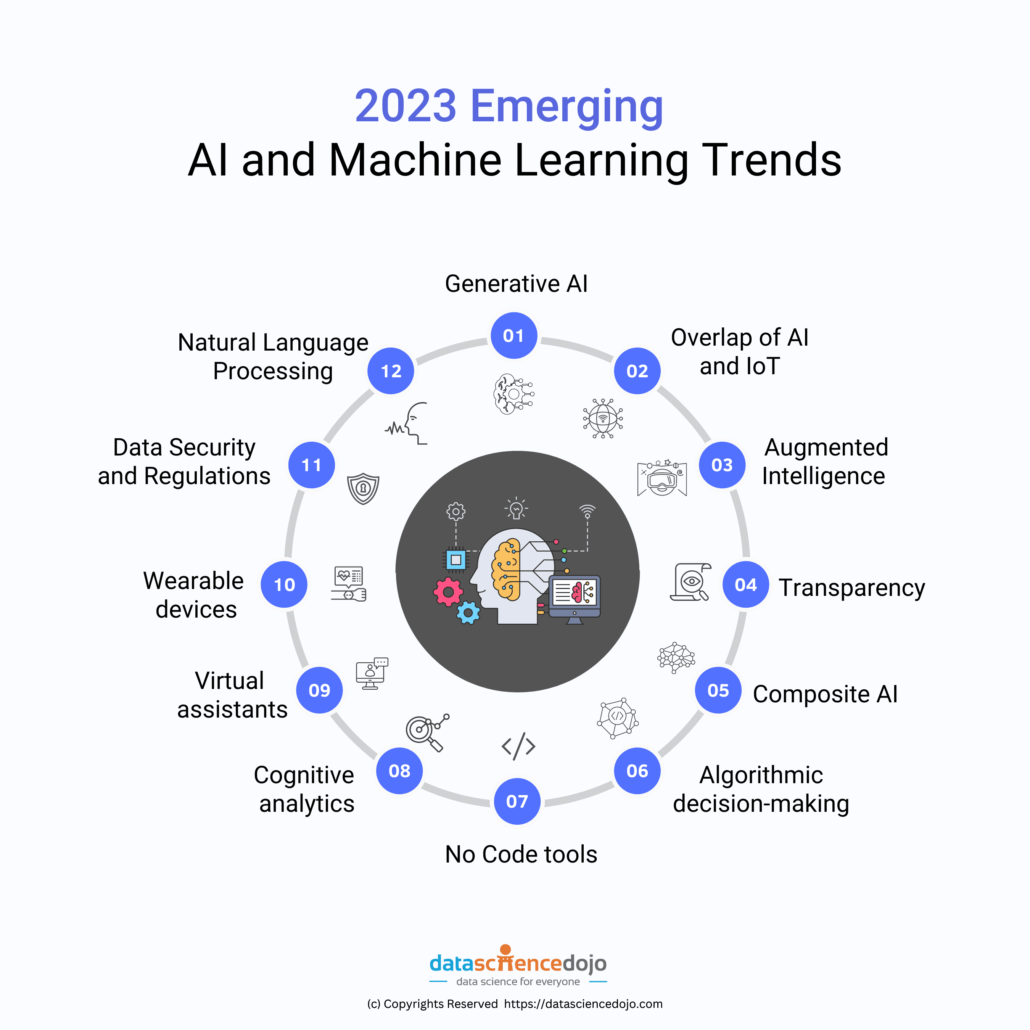
Table of Contents
Understanding I/O in AI Development
The Foundation of AI Interaction
In the context of AI, I/O (Input/Output) refers to the crucial process of data exchange between an AI system and its environment. This encompasses everything from feeding training data into a machine learning model (input) to receiving predictions, generating text, or controlling robotic actions (output). I/O is the lifeblood of any AI application, enabling it to learn, interact, and perform its intended tasks.
-
Examples of I/O in different AI applications:
- Image Recognition: Input: Images; Output: Classification labels (e.g., cat, dog, car).
- Natural Language Processing: Input: Text; Output: Translation, summarization, sentiment analysis.
- Robotics: Input: Sensor data (e.g., camera, lidar); Output: Motor commands, actions.
-
Data Formats and I/O Efficiency: The format of input and output data significantly impacts I/O efficiency. Efficient data formats like Parquet or Avro are crucial for optimizing processing speeds and reducing storage requirements. Poorly formatted data can lead to bottlenecks and slowdowns, hindering the overall performance of the AI system.
-
Optimized I/O for Fast and Reliable AI Performance: Optimized I/O is paramount for building fast and reliable AI systems. Techniques such as data pipelining, parallel processing, and specialized hardware (like GPUs and TPUs) are employed to accelerate data transfer and processing, enabling faster model training and inference. Efficient AI input/output is key to scaling AI solutions.
"io" and the Emerging AI Landscape
Deciphering the "io" Context
The term "io," in the context of AI, currently lacks a universally standardized definition. It might represent a specific project, library, or naming convention within the internal workings of companies like Google or OpenAI. It could potentially refer to a novel approach to I/O optimization or a specific component within a larger AI architecture, perhaps a new form of efficient data processing or a streamlined API interface. Further research is needed to definitively clarify its meaning in specific cases. It's possible "io" is simply used as shorthand within the community for input/output related work.
-
Examples of "io" Usage (Speculative): "io" might appear in internal documentation, project names, or codebases related to high-performance computing, or even the names of specific AI libraries handling input/output.
-
Potential Advantages and Disadvantages of the "io" Approach: Assuming "io" represents a novel technology, potential advantages could include improved efficiency, faster processing speeds, and reduced resource consumption. However, disadvantages might include compatibility issues, a lack of widespread adoption, or a steeper learning curve for developers.
-
Future Significance of "io": The long-term significance of "io" (if it represents a novel approach) will depend on its capabilities and community adoption. It could potentially revolutionize how we handle data in AI, impacting various aspects of AI development and deployment.
Google's Approach to I/O and AI
Google's I/O-centric Strategy
Google has made significant investments in AI, demonstrating a strong commitment to optimized I/O. Their approach is characterized by a focus on large-scale data processing and efficient data pipelines. They have developed a wide array of tools and services specifically designed for handling massive datasets and improving the efficiency of I/O operations.
-
Examples of Google's AI Products and Services: Google Cloud AI Platform, TensorFlow, and other Google AI services showcase strong I/O capabilities, enabling efficient model training and deployment at scale. Google's expertise in large-scale data processing is evident across many of its products.
-
Google's Focus on Large-Scale Data Processing: Google's ability to handle petabytes of data is a critical factor in its success. Their sophisticated infrastructure and optimized I/O techniques are essential for training the complex AI models that power many of their services.
-
Google-Specific Technologies Related to I/O Optimization: Google constantly develops and refines technologies to improve I/O efficiency in AI, including advancements in hardware (TPUs) and software frameworks like TensorFlow.
OpenAI's Strategies and the I/O/io Debate
OpenAI’s Focus (and potential use of "io")
OpenAI's approach to AI differs from Google's in several aspects. While Google focuses on building a broad ecosystem of AI tools and services, OpenAI is more focused on pushing the boundaries of AI capabilities, particularly in areas like reinforcement learning and generative AI. Whether or not they internally utilize a technology or shorthand labeled "io" remains unclear, but their focus on efficient model training and API access suggests sophisticated I/O management practices.
-
Examples of OpenAI's Key AI Projects: GPT-3, DALL-E, and other OpenAI models demonstrate impressive capabilities, hinting at sophisticated underlying architectures and likely significant investments in I/O optimization.
-
OpenAI's Emphasis on Specific AI Paradigms: OpenAI's emphasis on reinforcement learning and generative models necessitates efficient data handling and optimized I/O to train and deploy these complex models.
-
Differences in I/O Management Compared to Google: While both Google and OpenAI require efficient I/O, their approaches might differ based on their different priorities and the types of models they develop. Google's focus on scale contrasts with OpenAI's focus on achieving breakthrough capabilities.
The I/O vs. io Rivalry: Shaping the Future of AI
Impact on AI Development and Innovation
The competition between Google and OpenAI, and their differing approaches to I/O (or "io" if it emerges as a significant technology), drives innovation in the field. The quest for faster, more efficient, and more scalable AI systems pushes both organizations to constantly refine their methods, leading to breakthroughs in hardware, software, and data management techniques. This competition is fundamental to the rapid advancement of AI technology.
-
Potential Benefits of Different Approaches: Each approach – Google's focus on scalable infrastructure and OpenAI's on cutting-edge models – offers potential benefits, with each potentially paving the way for different advancements.
-
Potential Challenges and Limitations: Challenges include the development of efficient tools for each approach, ensuring compatibility, and addressing the need for specialized expertise.
-
Predictions for the Future Based on Current Trends: We can anticipate ongoing improvements in I/O efficiency and continued innovation in both infrastructure and model architectures. The "io" concept, if it gains traction, could become a pivotal element of this evolution.
Conclusion
The distinction between I/O and "io" in the AI landscape, while currently somewhat nebulous, highlights the intense competition driving advancements in artificial intelligence. Efficient AI input/output is undeniably critical for the success of any AI system. Google's focus on scalable infrastructure and OpenAI's emphasis on groundbreaking model development both represent crucial paths toward the future of AI. The ongoing evolution of I/O and related technologies promises continued breakthroughs, and understanding these dynamics is key to keeping pace with the field's rapid advancements. Learn more about the impact of I/O on AI, follow the ongoing I/O vs. io debate, and stay updated on the future of AI and the Google-OpenAI rivalry.
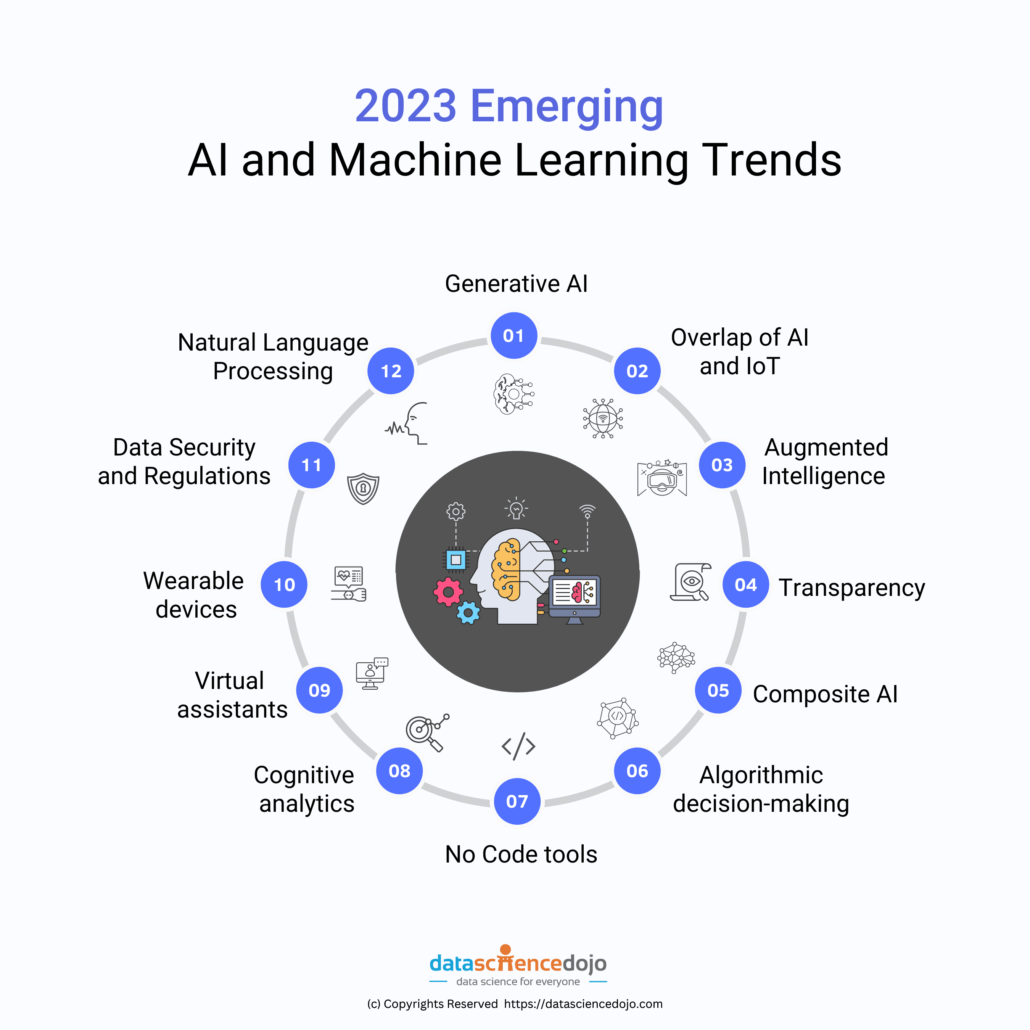
Featured Posts
-
Wta Italian Open Chinese Player Through To Quarters
May 26, 2025 -
Southern Vacation Destination Addresses Safety Concerns Following Shooting Incident
May 26, 2025 -
Flood Warnings And Flash Floods Your Guide To Safety And Preparedness
May 26, 2025 -
The Cinematic Glasgow How A Thriller Transforms The Citys Image
May 26, 2025 -
20 Anos Depois O Impacto Duradouro Do Trailer De Nome Do Filme
May 26, 2025
Latest Posts
-
Nba Lifts Ban John Haliburton Returns To Pacers Games
May 28, 2025 -
Promo Samsung Galaxy S25 Ultra 1 To 1294 90 E
May 28, 2025 -
Achetez Le Samsung Galaxy S25 Ultra 1 To Moins Cher
May 28, 2025 -
Bon Plan Smartphone Samsung Galaxy S25 Ultra 1 To
May 28, 2025 -
Offre Limitee Samsung Galaxy S25 Ultra 1 To A 13
May 28, 2025