Revolutionizing Drug Discovery: The Convergence Of Quantum Computing (QBTS) And AI
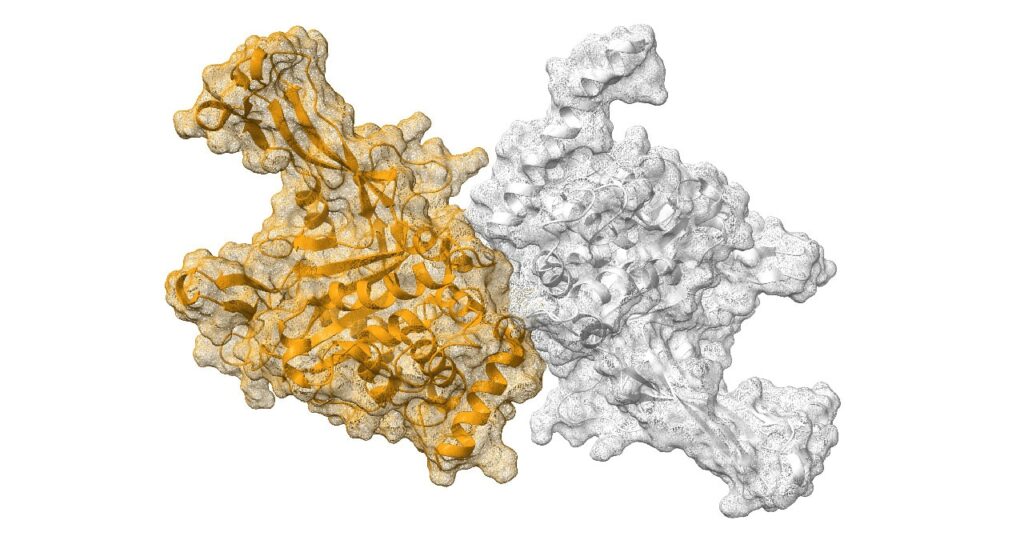
Table of Contents
Accelerating Drug Discovery with Quantum Computing (QBTS)
Quantum computing holds immense potential for accelerating various stages of drug discovery. Its unique capabilities allow for computational feats beyond the reach of classical computers.
Quantum Simulations for Molecular Modeling
QBTS significantly enhances our ability to simulate molecular interactions with unprecedented accuracy and speed. This capability is crucial because the efficacy and safety of a drug heavily depend on its interactions with biological targets at a molecular level.
- Faster calculations of molecular properties: QBTS allows for much faster calculation of crucial molecular properties like energy levels, dipole moments, and polarizability, which are essential for predicting drug behavior.
- Improved prediction of binding affinities: Accurate prediction of how strongly a drug molecule binds to its target is critical for assessing its efficacy. QBTS dramatically improves the precision of these predictions.
- Identification of potential drug candidates more efficiently: By simulating a vast number of molecular interactions, QBTS helps identify promising drug candidates far more efficiently than traditional methods.
Specific quantum algorithms, such as the Variational Quantum Eigensolver (VQE), are particularly useful for drug discovery. VQE enables the calculation of ground state energies of molecules, providing crucial information about their stability and reactivity. Successful applications of QBTS are already emerging in predicting protein folding and designing novel materials with specific therapeutic properties.
Optimizing Drug Design using Quantum Algorithms
Quantum algorithms are not limited to simulations; they can actively participate in the optimization of drug molecule design. This is crucial for fine-tuning drug properties to enhance efficacy and minimize side effects.
- Exploration of larger chemical spaces: Quantum algorithms allow researchers to explore a far larger chemical space than is computationally feasible with classical methods, potentially uncovering novel drug candidates with superior properties.
- Optimization of drug structures for better efficacy: By using techniques like the Quantum Approximate Optimization Algorithm (QAOA), researchers can optimize the structure of a drug molecule to maximize its binding affinity to the target while minimizing potential off-target effects.
- Identification of novel drug candidates: QBTS can assist in identifying novel chemical entities that would be difficult or impossible to discover using classical computational methods.
The application of QAOA and other quantum optimization algorithms holds immense promise in creating drugs with improved potency and reduced toxicity.
The Role of Artificial Intelligence (AI) in Drug Discovery
AI plays a crucial complementary role to QBTS, accelerating and enhancing various stages of the drug development pipeline. AI's ability to process and analyze massive datasets is invaluable in this field.
AI-driven Drug Target Identification
Identifying the right biological target is the first crucial step in drug development. AI algorithms can significantly accelerate this process by analyzing vast repositories of biological data.
- Faster identification of potential targets: AI algorithms can efficiently sift through genomic data, proteomic data, and other biological information to identify promising drug targets for various diseases.
- Improved accuracy in target selection: AI-powered predictive models can assess the likelihood of a target's success, reducing the risk of investing resources in less promising candidates.
- Reduction in research costs: By prioritizing targets with a higher probability of success, AI helps reduce wasted time and resources during the early stages of drug development.
Machine learning and deep learning techniques are particularly useful for drug target identification. These techniques can identify complex patterns and relationships in biological data that might otherwise go unnoticed.
AI-powered Drug Candidate Screening and Optimization
Once potential drug targets are identified, AI plays a vital role in screening and optimizing candidate molecules.
- Reduced experimental workload: AI can significantly reduce the need for expensive and time-consuming laboratory experiments by predicting the efficacy and safety of drug candidates in silico.
- Faster identification of lead compounds: AI algorithms can efficiently prioritize promising candidates from a large pool of potential drug molecules, accelerating the identification of lead compounds.
- Prediction of drug-drug interactions and adverse effects: AI models can predict potential drug-drug interactions and adverse effects, improving drug safety and reducing the risk of unexpected side effects.
AI applications in virtual screening, quantitative structure-activity relationship (QSAR) modeling, and generative models for drug design are proving increasingly valuable in accelerating this stage of drug development.
Synergistic Effects of QBTS and AI in Drug Discovery
The true power of revolutionizing drug discovery lies in the synergy between QBTS and AI. Combining these technologies creates a powerful engine for innovation.
Enhanced Predictive Capabilities
The integration of QBTS and AI leads to significantly improved predictive capabilities across various aspects of drug development.
- More accurate predictions: Combining the precise molecular simulations of QBTS with the data-driven insights of AI improves the accuracy of predictions related to drug efficacy, toxicity, and pharmacokinetics.
- Improved decision-making: More accurate predictions enable researchers to make better-informed decisions throughout the drug development pipeline, reducing the risk of failure and optimizing resource allocation.
- Reduced reliance on expensive and time-consuming experiments: By enhancing predictive power, the combined approach minimizes the reliance on expensive and time-consuming experimental validations.
Examples of integrated QBTS and AI workflows are already emerging, demonstrating the potential of this synergistic approach.
Accelerating the Drug Development Pipeline
The combined power of QBTS and AI has the potential to drastically shorten the drug development timeline.
- Faster time to market: The combined approach accelerates each stage of the drug development process, leading to faster access to new and improved treatments.
- Reduced overall development costs: By improving efficiency and reducing the need for extensive experimental validation, the combined approach reduces the overall cost of drug development.
- Quicker access to life-saving medications: Ultimately, this translates to quicker access to life-saving medications for patients in need.
Several pharmaceutical companies and research groups are actively exploring and implementing this combined approach, hinting at a future where drug development is significantly faster, more efficient, and more cost-effective.
Conclusion
Revolutionizing Drug Discovery: The Convergence of Quantum Computing (QBTS) and AI is no longer a futuristic vision; it's becoming a reality. The combined power of QBTS and AI offers unprecedented opportunities to accelerate drug discovery, enhancing accuracy, speed, and cost-effectiveness. The ability to perform highly accurate molecular simulations, coupled with AI's capacity to analyze vast datasets and predict drug behavior, is transforming the pharmaceutical landscape. The future holds immense promise for further advancements in this field, leading to new treatments for diseases that currently lack effective therapies. To stay informed about the latest advancements in this transformative field, explore further resources and research on the convergence of quantum computing and AI in drug discovery. Embrace the revolution and participate in shaping the future of healthcare.
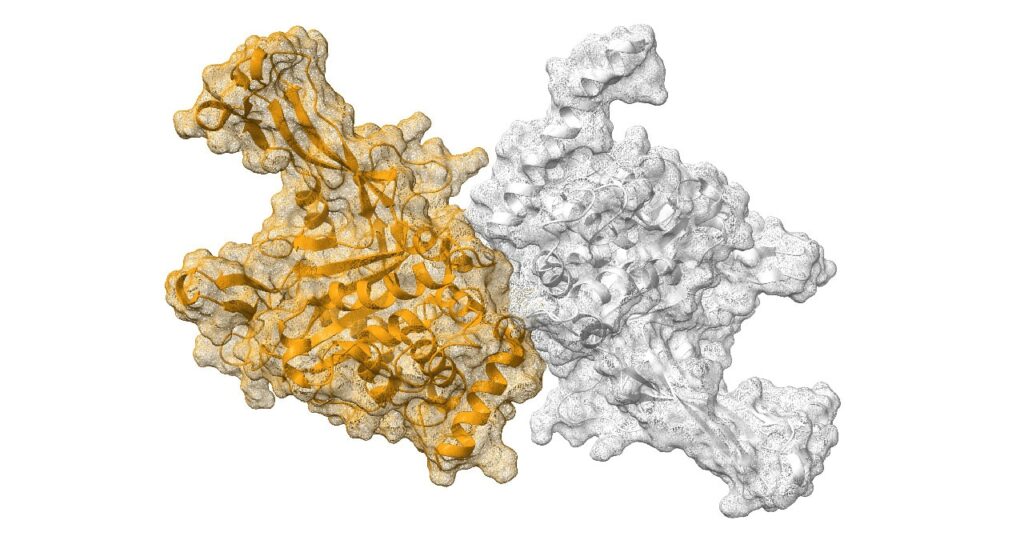
Featured Posts
-
The Goldbergs A Critical Analysis Of Its Success
May 21, 2025 -
Should You Invest In D Wave Quantum Qbts Stock Now
May 21, 2025 -
Novi Film Sydney Sweeney U Adaptaciji Reddit Price
May 21, 2025 -
New Images From Echo Valley Sydney Sweeney And Julianne Moores Upcoming Thriller
May 21, 2025 -
Porsches Dilemma A Ferrari Or A Mercedes The Impact Of Global Trade Conflicts
May 21, 2025
Latest Posts
-
Mia Wasikowska Cast In Taika Waititis Upcoming Family Movie
May 21, 2025 -
Watch Sandylands U The Ultimate Tv Schedule
May 21, 2025 -
Find Sandylands U On Tv Your Guide To Airtimes
May 21, 2025 -
Sandylands U Tv Listings And Showtimes
May 21, 2025 -
Gangsta Granny Comparing The Book To The Stage Adaptation
May 21, 2025