Turning "Poop" Into Profit: How AI Digests Repetitive Scatological Documents For Podcast Production
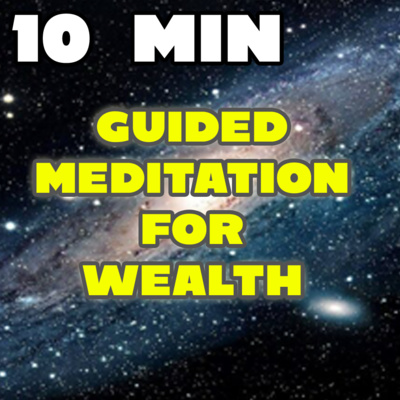
Table of Contents
H2: The Problem with Repetitive Data in Podcast Production
Podcast production often involves handling large amounts of repetitive data, significantly hindering efficiency and profitability. This tedious work directly impacts the bottom line.
H3: Time-Consuming Transcription and Editing
Manually transcribing and editing lengthy, repetitive audio or text documents is incredibly time-consuming and expensive. The cost of human labor adds up quickly, delaying podcast releases and impacting overall production schedules.
- Examples of repetitive data in podcasting:
- Medical case studies with repeated patient information.
- Legal transcripts filled with similar legal jargon and procedural details.
- Detailed scientific reports with redundant data points and experimental results.
- Impact of slow processing:
- Delayed podcast release schedules, leading to missed opportunities.
- Reduced overall production efficiency and increased operational costs.
- Potential loss of audience engagement due to delayed content delivery.
H2: AI-Powered Solutions for Data Digestion
Fortunately, AI offers powerful solutions to efficiently manage these repetitive data challenges. By harnessing the potential of AI, podcasters can significantly streamline their workflow and unlock hidden profit potential.
H3: Natural Language Processing (NLP) and its Role
Natural Language Processing (NLP) algorithms are crucial in understanding the context within repetitive documents. NLP allows AI to "read" and interpret human language, facilitating efficient data extraction and analysis.
- Specific examples of NLP tasks:
- Identifying key phrases and topics within lengthy transcripts.
- Summarizing lengthy documents into concise and informative summaries.
- Extracting relevant data points and statistics for analysis and reporting.
H3: Machine Learning for Pattern Recognition
Machine learning models excel at identifying patterns and anomalies within large datasets, automating many aspects of data analysis. This process significantly reduces manual effort and improves accuracy.
- Examples of machine learning applications:
- Automated data cleaning and pre-processing to remove irrelevant information.
- Identifying inconsistencies and errors within datasets for improved data quality.
- Flagging potential inaccuracies or anomalies for human review and verification.
H3: Automated Transcription and Summarization Tools
Several AI-powered tools are available to accelerate transcription and summarization. These tools significantly reduce the time and effort required to process large volumes of repetitive data.
- Examples of such tools:
- Otter.ai (offers real-time transcription and summarization).
- Descript (combines audio and video editing with transcription capabilities).
- Trint (provides accurate transcriptions and collaborative editing features). (Note: Always check for the most up-to-date and relevant tools.) These tools often boast features like speaker identification, timestamping, and export options tailored for various applications.
H2: Case Studies and Real-World Examples
The successful integration of AI into podcast production workflows has demonstrably improved efficiency and profitability.
H3: Success Stories
Several podcasts have already successfully utilized AI to handle repetitive data, resulting in quantifiable improvements.
- Examples of podcasts (hypothetical, replace with real-world examples if available):
- A medical podcast that uses AI to quickly summarize complex case studies, reducing editing time by 75%.
- A legal podcast that employs AI to extract key legal arguments from transcripts, improving research efficiency by 60%.
- A science podcast that uses AI to analyze research papers, generating concise summaries for each episode, freeing up researchers for content creation.
H3: Return on Investment (ROI)
The financial benefits of using AI for data processing are significant, leading to increased profitability and a faster return on investment.
- Examples of cost savings:
- Reduced labor costs associated with manual transcription and editing.
- Faster turnaround times, enabling more frequent podcast releases.
- Improved content quality due to more efficient data analysis and error reduction.
3. Conclusion:
Using AI to process repetitive data in podcast production offers substantial benefits, including significant time savings, substantial cost reductions, and improved overall efficiency. By efficiently managing the "poop" – the tedious, repetitive aspects of podcast production – you can focus your energy on creating high-quality content and building your audience. Start turning your "poop" into profit today! Explore AI-powered solutions and revolutionize your podcast production workflow, transforming repetitive documents into profitable podcasts and achieving efficient digestion of tedious data for profit.
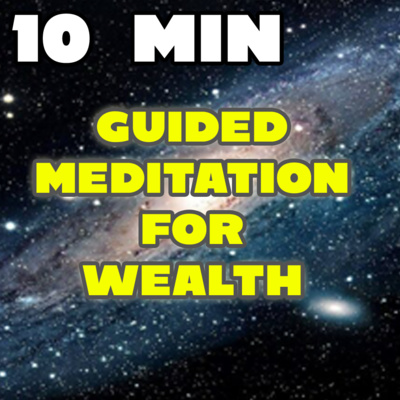
Featured Posts
-
Tasman Road Closure A Truckies Realistic Assessment And Call For Action
May 11, 2025 -
Muellers Future Post Bayern Transfer Speculation And League Preferences
May 11, 2025 -
Analyse Tactique Le Role De Mueller Dans La Victoire Du Bayern Contre L Inter
May 11, 2025 -
Tom Hanks And Tom Cruises 1 Debt The Story Behind The Unpaid Role
May 11, 2025 -
Medieval Book Cover Unveiling Merlin And Arthurs Hidden Tale
May 11, 2025
Latest Posts
-
Mtv Cribs Comparing The Homes Of Todays Wealthy Youth To Past Generations
May 12, 2025 -
Rich Kids Cribs A Tour Of Opulence And Excess On Mtv
May 12, 2025 -
Ru Pauls Drag Race S17 E13 Preview A Family Affair Drag Baby Mamas
May 12, 2025 -
Cbs Simulcast Of The Vmas A Sign Of Mtvs Decline
May 12, 2025 -
Watch 100 Mtv Unplugged Episodes Online Your Ultimate Streaming Guide
May 12, 2025